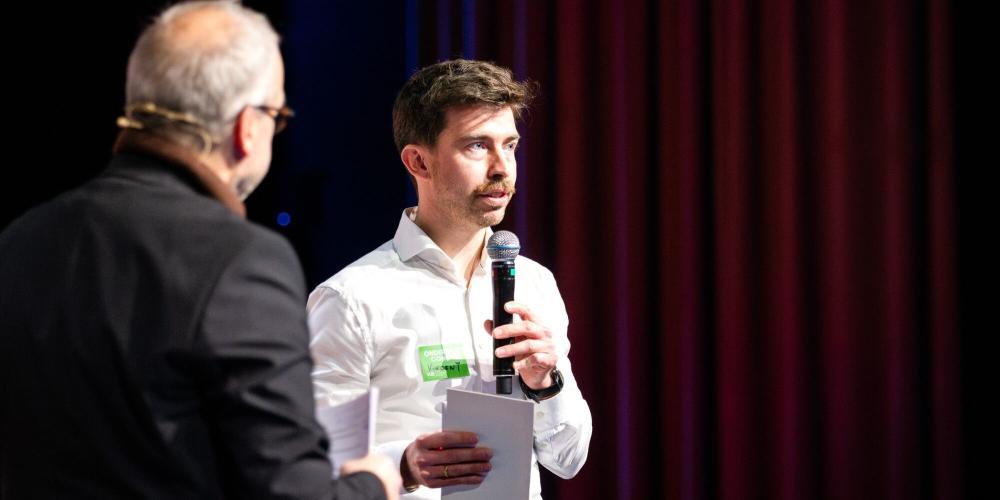
Vincent Ginis is assignment holder AI and Data at the VUB and helps shape the issue of ethical boundaries. A conversation about language theft, energy guzzlers and fraud. ‘Machine learning models also reinforce the biases present in society.’
“Things can change rapidly. I vividly recall how, during Caroline Pauwels’ rectorship, we were battling Covid-19, urgently shifting our operations to digital and online formats. Now, we can see that anything occurring behind a digital wall is more susceptible to fraud than what happens in real life. This presents a new area of tension. Many programmes are considering reverting to oral thesis defences and maximising in-person exams.”
What ethical boundaries should we be wary of?
“One boundary I personally focus on is human autonomy. There’s a potential danger in outsourcing many tasks and processes, leading to a loss of autonomy in performing them ourselves. This could extend to a loss of control over our own thoughts and outputs. In my view, this boundary is not sufficiently guarded.”
“It has become very easy to mimic people in the digital world, making it likely that individuals will inadvertently give up their privacy”
What remains of our privacy?
“In the short term, our privacy is significantly under pressure because, without intending to, we have all become part of the training material for models like language models. You’ve probably written many texts, a large portion of which have been noted by language models without your explicit consent. This is a loss of your privacy and it could have long-term consequences. It has become very easy to mimic people in the digital world, making it likely that individuals will inadvertently give up their privacy. In such a world, you would do everything to prove you are truly yourself. Some people already voluntarily have their irises scanned, for example.
“There’s also the dual use of new technology being employed in ways not originally intended. Consider the deployment of AI on the battlefield in Ukraine for large-scale drone attacks. Additionally, there’s an ecological impact that’s difficult to gauge fully right now.”
Could you guess at that impact?
“Currently, 40% of all computer computations are used for fundamental research, such as in healthcare, particle accelerators and pharmaceuticals. About 20% of computations today are for machine learning and AI. The projections for the computational needs of the next generation of generative pre-trained transformers, or GPTs, are staggering. It’s estimated that double-digit percentages of the world’s computers will be required to train just one GPT. This would also demand enormous amounts of energy, equivalent to that of at least one nuclear power plant, which we currently don’t have. And that’s not even considering the immense water usage for server cooling. People often talk about the energy consumption of bitcoins, but that’s insignificant compared to the large machine learning models on the horizon.”
“There are only a few models, and they will determine what is culturally accepted output for us”
“Machine learning models also reinforce societal biases. They are trained on existing data and reflect the patterns they find. For example, if you enter a sentence about a female doctor into Google Translate, the program may change it to a male doctor after double translation. Modern large language models add another layer of complexity. They not only learn patterns on the internet but reproduce them in a more refined manner. If you fact-check a culturally loaded statement on the internet, you might find less polished responses compared to what you get from ChatGPT. AI developers have added an extra step in ChatGPT called RLHF, or reinforcement learning from human feedback, which causes the models to reproduce their own cultural values. A few models will determine what is culturally accepted output.”
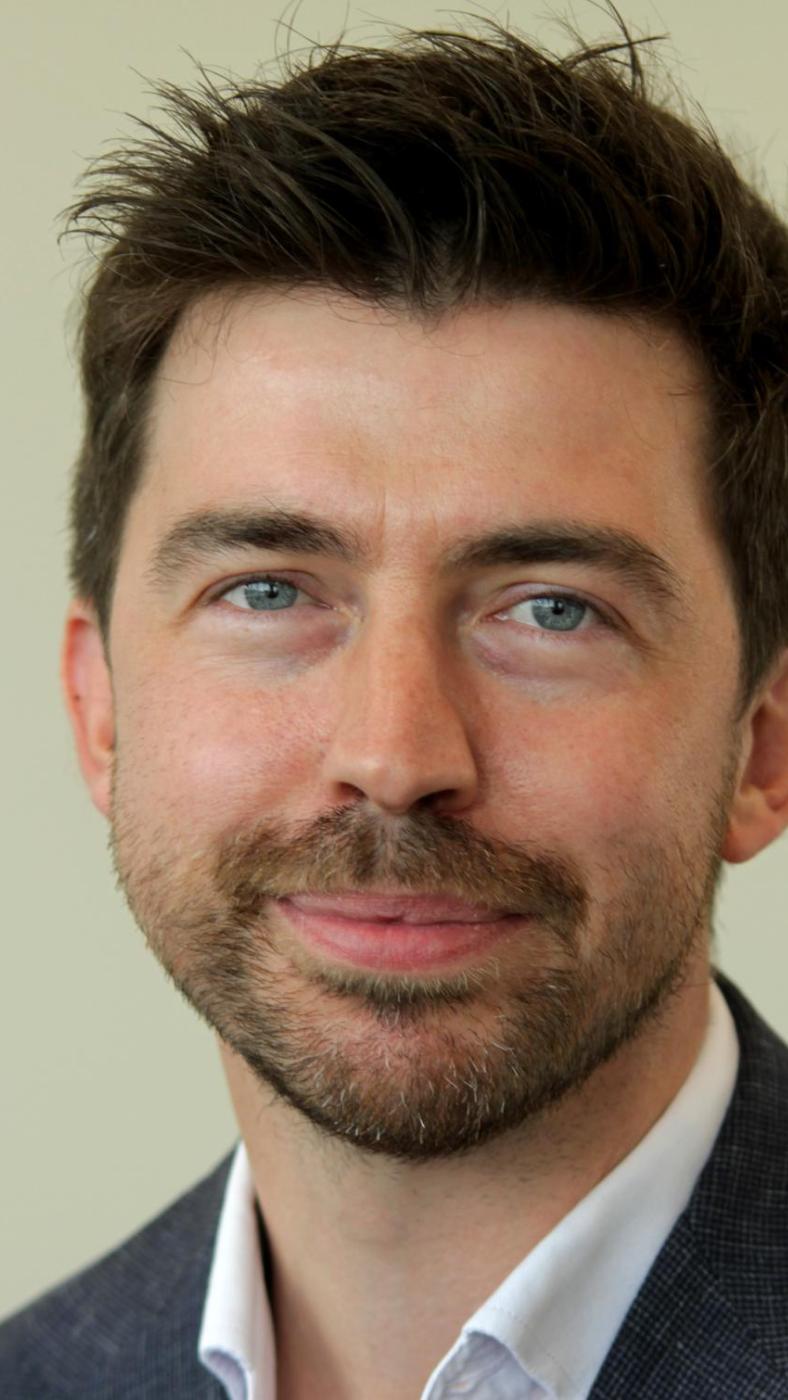
“We need to explain to our students when AI can be used. I compare it to the gym: you never see anyone taking a scooter onto the treadmill”
How should VUB handle AI?
“I want to highlight three areas: our strategic choices regarding education and research, and what we can contribute to society as a university. In education, we need to dispel two misconceptions. The first is that generative AI can only be used to write texts. It can also assist with non-text-based tasks. The second is that students no longer need to master the learning objectives that AI can accomplish. We need to talk to our students and explain when AI is and isn’t appropriate to use. I sometimes compare it to the gym: you never see anyone taking a scooter onto the treadmill. We understand in that situation that we have to go through a process to achieve our goals. We need to explain to students that sometimes they really have to stand on that treadmill alone. Making students owners of their learning trajectory is more important than ever.”
What does that look like in practice?
“Too many people ask AI to perform tasks for them. But you wouldn’t ask your brilliant colleague to write the introduction to your paper, would you? You’d discuss ideas and seek feedback. AI also has this conversational aspect, but it’s greatly underutilised. A problematic consequence is that if you outsource tasks for too long, you lose the skill yourself. The other danger is, of course, the ownership of material to which you haven’t contributed.”
Students’ theses are checked for originality. How does this verification mechanism work with GPT?
“Fraud isn’t new. There used to be an entire industry of ghostwriters for master’s theses, which has now collapsed. For €1,000, you’d get a thesis that Turnitin or similar programmes couldn’t check. And now? Students who used to commit fraud will continue to try. It’s crucial that we strengthen continuous process evaluation and use a variety of large and small measures to discourage fraud. But most students who worked responsibly in the past will continue to do so now, supported by examples of how to use language models positively.”